Law school graduate John Li knows every latest development of China’s autonomous driving industry—sort of.
The 24-year-old Hui Muslim from Guizhou—China’s budding big data industry center—has been working on data labeling tasks for autonomous vehicles for nearly two years. Li has witnessed the sensor technology for these driverless cars advancing from webcams to laser radars and now a combination of the two.
Li has yet to see a driverless car in person, but that doesn’t stop him from taking pride in his team’s work. He manages a team of about 100 “taggers” whose job mainly involves drawing boxes on pictures and video frames captured by cameras installed on driverless cars across the country, and then annotating the cars, bikes, pedestrians, and various traffic signs on those pictures and videos. These processed data then go into different datasets that are used to train artificial intelligence (AI) algorithms developed by China’s tech giants, like Alibaba, Baidu, and others.
“My work is to prepare clean data for autonomous driving, and I am making a small contribution to the technology’s advancement,” Li would often explain to friends and family who are intrigued by this new industry that found a base in the region just a few years ago.
Other than make annotations, Li and his colleagues sometimes handle other tasks like transcribing speeches in Chinese dialects (“I have heard dialects from every province and region”), annotating human and animal faces, and making TikTok videos of themselves dancing to music (the dance routines are choreographed ahead of time by unknown parties, so there are no freestyle surprises).
The Guangdong model
Li works in a data company tucked away in a small town surrounded by hills and mountains in Guizhou province, thousands of miles away from the country’s AI research and development heartlands of Beijing, Shanghai, Hangzhou, and Shenzhen.
It’s a template very similar to the Qiandian Houchang model—literally “front shop, back factory”—adopted by the manufacturing sector. In the late 1970s, companies in Hong Kong began to migrate their basic production and processing en masse to neighboring Guangdong province, turning what was at the time a poor, backward agricultural and fishing region with barely any industry of scale to the world’s factory. In 1978, Guangdong’s GDP was less than USD 13.5 billion. By 2018, that number eclipsed USD 1.47 trillion. (By comparison, Australia’s GDP in 2018 was USD 1.32 trillion.)
The recipe for Guangdong’s success is appealing to many inland provinces that have abundant labor force and limited industrialization. They see the potential of the data labeling industry—which is clean, labor-intensive, and supposedly high-tech—in generating tax revenue, providing stable employment, and facilitating the poverty alleviation campaign.
Guizhou, one of the poorest provinces in China, is now hoping to cash in on the rapid development of the country’s AI industry and related companies’ craving for relatively cheap and stable labor supply. The highland province set up a dedicated governmental department to oversee its data industry’s development and listed the promotion of data services—including data labeling, collection, and other data processing services—as one of the priority tasks for this year.
The municipal government of the Buyi and Miao Autonomous Prefecture of Qiannan, where Li’s company is based, has set up an RMB 30 million fund dedicated to the promotion of local big data industries in 2018. Companies registered in the region are entitled to various government subsidies for rent, broadband, and electricity for three years, as well as generous tax breaks for five years.
Xia Bingqing, a scholar researching China’s digital economy at East China Normal University, noticed the local government’s effort to reduce poverty by introducing and supporting the data labeling industry when she was conducting fieldwork in Guizhou. The governmental push for the data labeling industry could be a supportive policy for the local villages and alleviate poverty if everything goes well, she said.
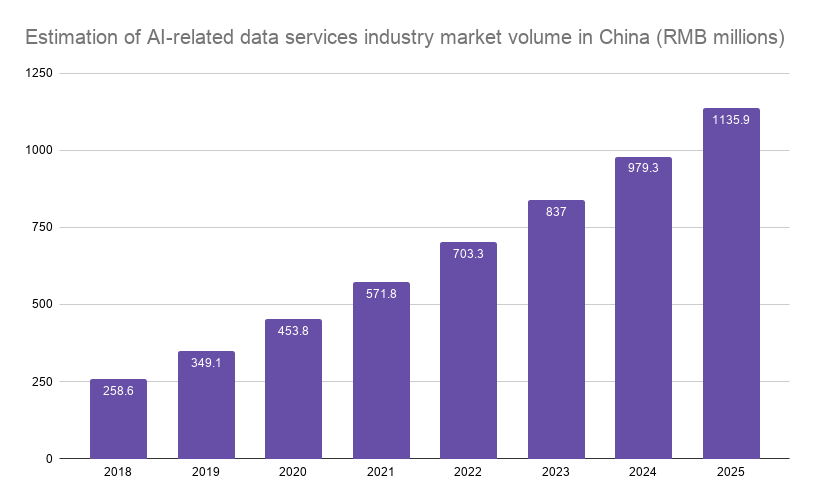
A fleeting opportunity
Guizhou is not alone in embracing the data labeling industry.
Shanxi, famed for its wealth in coal deposits, wants to bring in more than 100 data labeling companies and train more than 10,000 workers by 2022. The mining province aims to be the leading powerhouse for China’s data sector and have an RMB 5 billion industry by 2025.
While these local governments are appealing to the AI industry’s pursuit of lower production costs by providing sound infrastructure, generous government incentives, and cheap labor, the AI industry responds by attempting to accommodate local officials’ desire for stable employment and an improved standard of living.
Alipay Foundation and Alibaba AI labs launched its “A-Idol Initiative,” which provides free training courses on labeling and curating data for women in the country’s vast underdeveloped areas. The initiative, which started out in Guizhou’s Tongren city, where more than 580,000 locals are currently living below the poverty line, will expand to cover ten poverty-stricken counties across China. Alibaba AI Labs, the AI research arm of Chinese tech giant Alibaba, also committed to making at least RMB 10 million data processing orders annually to ensure the sustainability of the initiative.
But data labeling initiatives with heavy tech industry backing and guaranteed orders are few and far between, industry insiders observed. Most local data labeling pushes in the less developed areas are facing an unavoidable sustainability problem—as machine learning progresses, data labeling tasks will become harder and harder, with contracts shrinking.
“For our industry, expertise is very important. Those so-called data villages don’t have the expertise. They think they can handle data as long as they can use computers, but they will find their [data labeling] tasks getting more and more difficult,” said Du Lin, CEO of Beijing-based data processing startup Basic Finder. Though data labeling, like other business processes that are outsourced, is labor-intensive, the current push for lower labor cost is taking the industry in the wrong direction, he said.
Du’s company has insisted on having its own data centers in and around Beijing rather than moving them to places where the labor costs are cheaper. “Though that would mean higher costs, we think in the long run we can train our staff and have an edge in our core competitiveness.”
Basic Finder has turned down invitations from local governments to set up operations elsewhere and is about to launch a new version of the crowdsourcing platform they purchased last year. “If we were to set up cooperation with the local governments, we must make sure there are enough tasks for those local industries. But at the moment, we find that most of our demands could be solved by crowdsourcing because it’s more efficient,” Du said. “There’s no need for the dedicated workforce.”
A data industry insider who goes by the pseudonym Seven and once managed a nearly 8,000-member freelance data labeling online collaboration team, agreed with Du’s reluctance to shift data labeling jobs to less developed regions. “Unless big companies support them and keep feeding them orders, or state-owned enterprises take over and look after the staff, they will find themselves unable to support their operation because of the declining orders,” he said.
Maturing fast
Changes in the data labeling industry are happening so fast that it has gone through several stages of the industry life cycle—introduction and growth—which for other industries took decades. At the peak of demand for speech data labeling in 2017 (partly due to the proliferation of smart speakers), sometimes three to four thousand people from Seven’s team would work on the same task together. But since the second half of last year, those tasks have been disappearing from major crowdsourcing platforms. “Once a mature product is out, there won’t be much need for speech data labeling tasks,” he said. “It’s like digging our own graves.”
Seven has disbanded his freelancer collaboration team, as did many other smaller data labeling teams scattered in China’s low-tier cities and rural areas. John Li plans to move on to other endeavors in the next few years.
Basic Finder’s Du sees the silver lining in the rapid shakeup—the industry is weeding out weak players and those who remain in the game are becoming stronger. “It’s unavoidable, and it’s a good trend,” he said. “This forces the industry to become more professional.”